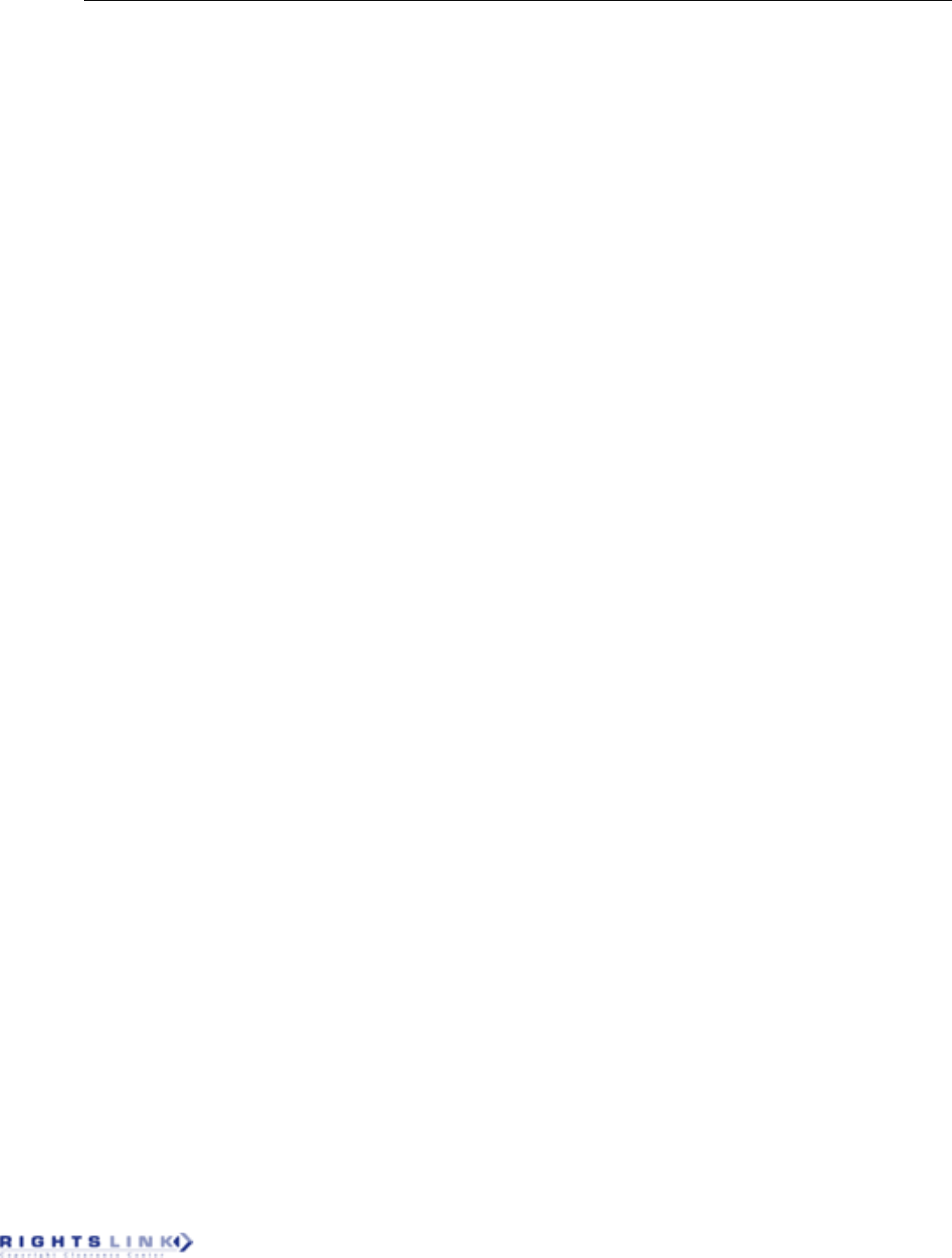
Honka and Chintagunta: Search Strategies in the U.S. Auto Insurance Industry
Marketing Science 36(1), pp. 21–42, © 2017 INFORMS 41
to estimating the marketwide distributions of insurance prices and
does not suffer from a selection bias.
21
Note that because of the size of our data, we are not able to
include company-specific effects of demographic variables in con-
sumers’ price expectations.
22
Details on these psychographic factors and summary statistics of
the items that constitute them are available from the authors on
request.
23
The technical reason for the necessity of an additional assumption
is as follows: If consumers do not optimally choose the company to
search first, we cannot derive a general mathematical representation
of the proportion of below-price-expectation actual prices among
consumers who do not search beyond the first quote without addi-
tional information/data or other additional assumptions—neither
under simultaneous nor sequential search (we provide details why
this is the case in Sections 5.3.1 and 5.3.2).
24
This applies to differentiated goods. We view and model auto
insurance as a differentiated good.
25
Consumers stop searching and purchase when the maximum
reservation utility among the searched companies is larger than the
maximum reservation utility among the nonsearched companies
(Weitzman 1979).
26
Note that the necessary condition that we observe consumers to
search more than once is satisfied in our data.
27
Recall that insurance prices depend on consumer and policy char-
acteristics. In estimating the distribution of prices, we account for
this by making the expected price a function of these consumer
and policy characteristics. Thus, when calculating the proportion of
below-expectation prices, we compare actual prices in consumers’
consideration sets to consumer- and company-specific expected
prices.
28
We calculate search costs in dollars by dividing the search cost
coefficient c by the price coefficient β
1
.
29
We also estimated a sequential search model in which search
costs are a function of company-specific advertising spending in the
month prior to the consumer’s insurance purchase. While advertis-
ing spending significantly decreases search costs, the log-likelihood
of such a sequential search model remains worse than the log-
likelihood of the simultaneous search model reported in column (i)
in Table 5. Note that we cannot estimate such a model under simul-
taneous search, as search costs cannot be company-specific in our
estimation approach (Chade and Smith 2005).
30
Morgan and Manning (1985) find that using simultaneous search
is more efficient for consumers when prices need to be gathered
quickly. Chade and Smith (2006) and Kircher (2009) find that using
simultaneous search is more efficient for consumers when the other
side of the market might reject the consumer. Variables that translate
into these two factors in the auto insurance market are potentially
the timing of the price search process (close to the policy expiration
date or weeks in advance), tickets and accidents in the past, and
low credit scores.
References
Andrews R, Srinivasan T (1995) Studying consideration effects in
empirical choice models using scanner panel data. J. Marketing
Res. 32(1):30–41.
Baye M, Morgan J, Scholten P (2006) Information, search, and price
dispersion. Hendershott T, ed. Handbook on Economics and Infor-
mation Systems (Elsevier, Amsterdam), 323–376.
Benabou R (1993) Search market equilibrium, bilateral heterogene-
ity, and repeat purchases. J. Econom. Theory 60(1):140–158.
Bronnenberg B, Vanhonacker W (1996) Limited choice sets, local
price response, and implied measures of price competition.
J. Marketing Res. 33(2):163–173.
Burdett K, Judd K (1983) Equilibrium price dispersion. Econometrica
51(4):955–970.
Chade H, Smith L (2005) Simultaneous search. Working paper, Yale
University, New Haven, CT.
Chade H, Smith L (2006) Simultaneous search. Econometrica
74(5):1293–1307.
Chen X, Hong H, Shum M (2007) Nonparametric likelihood
ratio model selection tests between parametric likelihood and
moment condition models. J. Econometrics 141(1):109–140.
Chen Y, Yao S (2016) Sequential search with refinement: Model
and application with click-stream data. Management Sci.
Forthcoming.
Chiang J, Chib S, Narasimhan C (1999) Markov chain Monte Carlo
and models of consideration set and parameter heterogeneity.
J. Econometrics 89(1–2):223–248.
Dahlby B, West D (1986) Price dispersion in an automobile insur-
ance market. J. Political Econom. 94(2):418–438.
Dayton C, Macready G (1988) Concomitant-variable latent-class
models. J. Amer. Statist. Assoc. 83(401):173–178.
De los Santos B, Hortaçsu A, Wildenbeest M (2012) Testing models
of consumer search using data on Web browsing and purchas-
ing behavior. Amer. Econom. Rev. 102(1):2455–2480.
De los Santos B, Hortaçsu A, Wildenbeest M (2015) Search with
learning. J. Bus. Econom. Statist. Forthcoming.
DeSarbo W, Jedidi K (1995) The spatial representation of heteroge-
neous consideration sets. Marketing Sci. 14(3):326–342.
DeSarbo W, Lehman D, Carpenter G, Sinha I (1996) A stochastic
multidimensional unfolding approach for representing phased
decision outcomes. Psychometrika 61(3):485–508.
Gensch D, Soofi E (1995) Information-theoretic estimation of indi-
vidual consideration set. Internat. J. Res. Marketing 12(1):25–38.
Guadagni P, Little J (1983) A logit model of brand choice. Marketing
Sci. 2(2):203–238.
Gumbel EJ (1961) Bivariate logistic distributions. J. Amer. Statist.
Assoc. 56(294):335–349.
Hajivassiliou V (2000) Some practical issues in maximum simu-
lated likelihood. Mariano R, Schuermann T, Weeks M, eds.
Simulation-Based Inference in Econometrics: Methods and Applica-
tions (Cambridge University Press, Cambridge, UK), 71–99.
Hauser J, Wernerfelt B (1990) An evaluation cost model of consid-
eration sets. J. Consumer Res. 16(4):393–408.
Hong H, Shum M (2006) Using price distributions to estimate search
costs. RAND J. Econom. 37(2):257–275.
Honka E (2014) Quantifying search and switching costs in the U.S.
auto insurance industry. RAND J. Econom. 45(4):847–884.
Honka E, Hortaçsu A, Vitorino M (2016) Advertising, consumer
awareness, and choice: Evidence from the U.S. banking indus-
try. Working paper, University of California Los Angeles.
Janssen M, Moraga-Gonzalez J, Wildenbeest M (2005) Truly costly
sequential search and oligopolistic pricing. Internat. J. Indust.
Organ. 23(5):451–466.
Kamakura W, Russell G (1989) A probabilistic choice model for
market segmentation and elasticity structure. J. Marketing Res.
26(4):379–390.
Kim J, Albuquerque P, Bronnenberg B (2010) Online demand under
limited consumer search. Marketing Sci. 29(6):1001–1023.
Kircher P (2009) Efficiency of simultaneous search. J. Political
Econom. 117(5):861–913.
Lin H, Wildenbeest M (2015) Search and prices in the Medi-
gap insurance market. Working paper, Indiana University,
Bloomington.
Matsumoto B, Spence F (2014) Price beliefs and experience: Do con-
sumers’ beliefs converge to empirical distributions with repeat
purchases? Working paper, University of North Carolina at
Chapel Hill, Chapel Hill.
McFadden D (1986) The choice theory approach to market research.
Marketing Sci. 5(4):275–297.